The Automatic Joint Teeth Segmentation in Panoramic Dental Images using Mask Recurrent Convolutional Neural Networks with Residual Feature Extraction:
Can it be useful in Oral Cancer Diagnosis and Management?
DOI:
https://doi.org/10.59667/sjoranm.v12i1.18Keywords:
Medical image segmentation, Image segmentation using MRCNN Medical Imaging, Teeth segmentation, AI in Oral Cancer, CNN in Oral CancerAbstract
Introduction
Panoramic dental images gives an in-depth understanding of the tooth structure, both lower and upper jaws, and surrounding structures throughout the cavity in our mouth.The Panoramic dental images provided have significance for dental diagnostics since they aid in the detection of an array of dental disorders, including oral cancer. We propose a novel approach to automatic joint teeth segmentation using the pioneer Mask Recurrent Convolutional Neural Network (MRCNN) model for dental image segmentation.
Material and Methods
In this study, a sequence of residual blocks are used to construct a 62-layer feature extraction network in lieu of ResNet50/101 in MRCNN. To evaluate the efficacy of our method, the UFBA-UESC and Tufts dental image dataset (2500 panoramic dental x-rays) were utilised. 252 x-rays were used in test set, rest of the x-rays were utilised as training(1800 images) and validation datasets(448images) in ratio of 8:2 of the modified MRCNN model.
Results
Modified MRCNN achieved the final training and validation accuracies as 99.67% and 98.94%, respectively.The achieved accuracy of Dice coefficient (97.8%), Intersection over Union, (98.67%), and Pixel Accuracy(96.53%) respectively over the whole dataset. We also compare the performance of proposed model and other well established networks such as FPN, UNet, PSPNet, and DeepLabV3. The Modified MRCNN provides better results segmenting any two teeth which are close.
Conclusion
Our proposed method will serve as a valuable tool for automatic segmentation of individual teeth for medical management. This current method leads to higher accuracy and precision. Segmented images can be used to evaluate periodic changes, providing valuable data for assessing the progression of oral cancer and the efficacy of management.Future research should focus on developing less complex, lightweight, and faster vision models while maintaining high accuracy.
References
1. G. Silva, L. Oliveira, and M. Pithon, “Automatic segmenting teeth in x-ray images: Trends, a novel data set, benchmarking and future perspectives,” Expert Systems with Applications, vol. 107, pp. 15 – 31, 2018. doi: https://doi.org/10.1016/j.eswa.2018.04.001
2. A. Lurie, G. M. Tosoni, J. Tsimikas, and W. Fitz, “Recursive hierarchic segmentation analysis of bone mineral density changes on digital panoramic images,” Oral Surgery, Oral Medicine, Oral Pathology and Oral Radiology, vol. 113(4), pp. 549–558, 2012. https://doi.org/10.1016/j.oooo.2011.10.002
3. Y.Y. Amer and M. J. Aqel, “An efficient segmentation algorithm for panoramic dental images,” Procedia Computer Science, vol. 65, pp. 718–725, 2015, international Conference on Communications, management, and Information technology (ICCMIT’2015). https://doi.org/10.1016/j.procs.2015.09.016
4. M. K. Alsmadi, “A hybrid fuzzy c-means and neutrosophic for jaw lesions segmentation,” Ain Shams Engineering Journal, vol. 9, no. 4, pp. 697–706, 2018. https://doi.org/10.1016/j.asej.2016.03.016
5. M. R. M. Razali, N. S. Ahmad, R. Hassan, Z. M. Zaki, and W. Ismail, “Sobel and canny edges segmentations for the dental age assessment,” in 2014 International Conference on Computer Assisted System in Health, 2014, pp. 62–66. https://doi.org/10.1109/CASH.2014.10
6. G. Jader, J. Fontineli, M. Ruiz, K. Abdalla, M. Pithon, and L. Oliveira, “Deep instance segmentation of teeth in panoramic x-ray images,” in 2018 31st SIBGRAPI Conference on Graphics, Patterns and Images (SIBGRAPI), 2018, pp. 400–407. https://doi.org/10.1109/SIBGRAPI.2018.00058
7. K. He, G. Gkioxari, P. Dollar, and R. Girshick, “Mask R-CNN,” in ´ 2017 IEEE International Conference on Computer Vision (ICCV), 2017, pp.2980–2988.
8. T. L. Koch, M. Perslev, C. Igel, and S. S. Brandt, “Accurate segmentation of dental panoramic radiographs with U-Nets,” in 2019 IEEE 16th International Symposium on Biomedical Imaging (ISBI 2019), 2019, pp. 15–19. https://doi.org/10.1109/ISBI.2019.8759563
9. O. Ronneberger, P.Fischer, and T. Brox, “U-net: Convolutional networks for biomedical image segmentation,” in Medical Image Computing and Computer-Assisted Intervention (MICCAI), ser. LNCS, vol. 9351. Springer, 2015, pp. 234–241. https://doi.org/10.1007/978-3-319-24574-4_28
10. R. Girshick, J. Donahue, T. Darrell, and J. Malik, “Region-Based Convolutional Networks for Accu-rate Object Detection and Segmentation” IEEE Transactions on Pattern Analysis and Machine In-telligence, vol. 38, no. 1, pp. 142–158, Jan. 2016, https://doi.org/10.1109/TPAMI.2015.2437384
11. R. Girshick, “Fast R-CNN,” in Proceedings of the IEEE International Conference on Computer Vision, 2015, pp. 1440–1448. https://doi.org/10.1109/ICCV.2015.169
12. S. Ren, K. He, R. Girshick, and J. Sun, “Faster R-CNN: Towards Real-Time Object Detection with Region Proposal Networks”, IEEE Transactions on Pattern Analysis and Machine Intelligence, vol. 39, no. 6, pp. 1137–1149, Jun. 2017. https://doi.org/10.1109/TPAMI.2016.2577031
13. T.-Y. Lin, P. Dollár, R. Girshick, K. He, B. Hariharan, and S. Belongie, “Feature Pyramid Networks for Object Detection”, in Proceedings of the IEEE Conference on Computer Vision and Pattern Recognition (CVPR), 2017, pp. 2117–2125. https://doi.org/10.1109/CVPR.2017.106
14. F. Deng, H. Hu, S. Chen, Q. Guan, and Y. Zou, “Rich feature hierarchies for cell detecting under phase contrast microscopy images”, in 2015 Sixth International Conference on Intelligent Control and Information Processing (ICICIP), Nov. 2015, pp. 348–353. https://doi.org/10.1109/ICICIP.2015.7388195
15. N. Atif, M. Bhuyan, and S. Ahamed, “A Review on Semantic Segmentation from a Modern Pers-pective,” in 2019 International Conference on Electrical, Electronics and Computer Engineering (UPCON), Nov. 2019, pp. 1–6. https://doi.org/10.1109/UPCON47278.2019.8980189
16. Simonyan, K., Zisserman, A.: Very deep convo-lutional networks for large-scale image recognition. arXiv preprint arXiv:1409.1556 (2014)https://doi.org/10.48550/arXiv.1409.1556
17. Szegedy, C., Liu, W., Jia, Y., Sermanet, P., Reed, S., Anguelov, D., Erhan, D., Vanhoucke, V., Rabinovich, A.: Going deeper with convolutions. In: Proceedings of the IEEE Conference on Computer Vision and Pattern Recognition, pp. 1–9 (2015) https://www.cv-foundation.org/openaccess/content_cvpr_2015/html/Szegedy_Going_Deeper_With_2015_CVPR_paper.html
18. He, K., Zhang, X., Ren, S., Sun, J.: Deep residual learning for image recognition. In: Proceedings of the IEEE Conference on Computer Vision and Pattern Recognition, pp. 770–778 (2016) https://openaccess.thecvf.com/content_cvpr_2016/html/He_Deep_Residual_Learning_CVPR_2016_paper.html
19. K. Panetta, R. Rajendran, A. Ramesh, S. P. Rao and S. Agaian, "Tufts Dental Database: A Multimodal Panoramic X-Ray Dataset for Benchmarking Diagnostic Systems", in IEEE Journal of Biomedical and Health Informatics, vol. 26, no. 4, pp. 1650-1659, April 2022, https://doi.org/10.1109/JBHI.2021.3117575
20. Mask-RCNN implementation for Tensorflow 2.7.0 and Keras 2.7.0. https://github.com/Kamlesh364/Mask-RCNN-TF2.7.0-keras2.7.0
21. Z. Zhou, M. M. R. Siddiquee, N. Tajbakhsh, and J. Liang, “Unet++: A nested U-net architecture for medical image segmentation,” in Deep Learning in Medical Image Analysis and Multimodal Learning for Clinical Decision Support, Berlin, Germany: Springer, 2018, pp. 3–11. https://doi.org/10.1007/978-3-030-00889-5_1
22. H. Zhao, J. Shi, X. Qi, X. Wang, and J. Jia, “Pyramid scene parsing network,” in Proc. IEEE Conf. Comput. Vis. Pattern Recognit., 2017, pp. 2881–2890. https://openaccess.thecvf.com/content_cvpr_2017/html/Zhao_Pyramid_Scene_Parsing_CVPR_2017_paper.html
23. L.-C. Chen, G. Papandreou, F. Schroff, and H. Adam, “Rethinking atrous convolution for semantic image segmentation,” 2017, arXiv:1706.05587.
24. L.-C. Chen, Y. Zhu, G. Papandreou, F. Schroff, and H. Adam, “Encoderdecoder with atrous separable convolution for semantic image segmentation,” in Proc. Eur. Conf. Comput. Vis., 2018, pp. 801–818. https://openaccess.thecvf.com/content_ECCV_2018/html/Liang-Chieh_Chen_Encoder-Decoder_with_Atrous_ECCV_2018_paper.html
25. Su, B., Zhang, Q., Gong, Y. et al. Deep learning-based classification and segmentation for scalpels. Int J CARS (2023). https://doi.org/10.1007/s11548-022-02825-7
26. Wang, H., Xiao, N., Luo, S. et al. Multi-scale dense selective network based on border modeling for lung nodule segmentation. Int J CARS (2023). https://doi.org/10.1007/s11548-022-02817-7
27. Manjunatha, Y., Sharma, V., Iwahori, Y. et al. Lymph node detection in CT scans using modified U-Net with residual learning and 3D deep network. Int J CARS (2023). https://doi.org/10.1007/s11548-022-02822-w
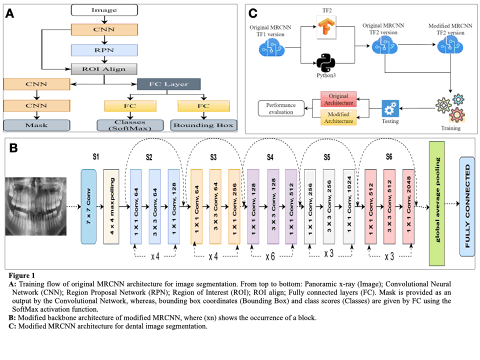
Downloads
Published
License
Copyright (c) 2024 Raghavendra H. Bhalerao, Abhijeet Ashok Salunke, Shristi Sharan, Kamlesh Kumar, Priyank Rathod, Prince Kumar, Manish Chaturvedi, Nandlal Bharwani, Krupa Shah, Dhruv Patel, Keval Patel, Vikas Warikoo, Manisha Abhijeet Salunke, Shashank Pandya

This work is licensed under a Creative Commons Attribution 4.0 International License.
This license requires that reusers give credit to the creator. It allows reusers to distribute, remix, adapt, and build upon the material in any medium or format, even for commercial purposes.