Supporting Radiologists with Automated Image Analysis: An Evaluation of Deep Learning Tools for Augmenting Breast Cancer Screening
DOI:
https://doi.org/10.59667/sjoranm.v18i1.16Keywords:
Artificial Neural Networks (ANNs), Predictive analytics, Breast cancer, Risk factors, Personalized preventionAbstract
Introduction:
This study investigates the use of Artificial Neural Networks (ANNs) for analyzing breast cancer risk factors, aiming to improve predictive analytics in early diagnosis and prevention. By focusing on complex patterns among genetic, hormonal, lifestyle, and environmental factors, the objective is to determine how effectively ANNs can rank and assess these risks.
Methodology:
ANNs were applied to large datasets containing patient histories, medical records, and genetic information to evaluate their predictive power. The study leveraged deep learning techniques to process intricate, nonlinear relationships that traditional statistical approaches may overlook. Risk factors were analyzed to identify significant patterns, and the ANNs were tuned to optimize prediction accuracy and reliability.
Results and Discussion:
The results showed that ANNs could successfully identify key risk factors for breast cancer and rank them based on predictive strength. Deep learning techniques enhanced the accuracy of predictions, revealing subtle, nonlinear correlations among risk factors. However, challenges were noted in interpreting neural network models due to their complexity, and limitations in data quality and balance impacted outcomes. These findings highlight the advantages of ANNs in personalized risk assessment but emphasize the need for continued refinement to address interpretability issues.
Conclusion:
ANNs demonstrate considerable potential to advance breast cancer risk prediction, offering valuable insights for personalized prevention strategies. While further work is needed to optimize these models and integrate them effectively into clinical practice, ANNs could significantly enhance early risk assessment and improve outcomes in breast cancer.
References
1. World Health Organization. (2021). Breast cancer: Key facts. Retrieved from https://www.who.int/news-room/fact-sheets/detail/breast-cancer
2. Momenimovahed, Z., & Salehiniya, H. (2019). Epidemiological characteristics of and risk factors for breast cancer in the world. Breast Cancer: Targets and Therapy, 11, 151-164. https://doi.org/10.2147/BCTT.S176070
3. Rabiei R, Ayyoubzadeh SM, Sohrabei S, Esmaeili M, Atashi A. Prediction of Breast Cancer using Machine Learning Approaches. J Biomed Phys Eng. 2022 Jun 1;12(3):297-308. PMID: 35698545; PMCID: PMC9175124. https://doi.org/10.31661/jbpe.v0i0.2109-1403
4. Esteva, A., Robicquet, A., Ramsundar, B., Kuleshov, V., DePristo, M., Chou, K., ... & Dean, J. (2019). A guide to deep learning in healthcare. Nature Medicine, 25(1), 24-29. https://doi.org/10.1038/s41591-018-0316-z
5. LeCun, Y., Bengio, Y., & Hinton, G. (2015). Deep learning. Nature, 521 (7553), 436-444. https://doi.org/10.1038/nature14539
6. Uchikov P, Khalid U, Dedaj-Salad GH, Ghale D, Rajadurai H, Kraeva M, Kraev K, Hristov B, Doykov M, Mitova V, Bozhkova M, Markov S, Stanchev P. (2024). Artificial Intelligence in Breast Cancer Diagnosis and Treatment: Advances in Imaging, Pathology, and Personalized Care. Life (Basel). 8;14(11):1451. PMID: 39598249; PMCID: PMC11595975. https://doi.org/10.3390/life14111451
7. Manali, D., Demirel, H., Eleyan, A. (2024). Deep Learning Based Breast Cancer Detection Using Decision Fusion. Computers, 13, 294. https://doi.org/10.3390/computers13110294
8. Korica, P., Gayar, N.E., Pang, W. (2021). Explainable Artificial Intelligence in Healthcare: Opportunities, Gaps and Challenges and a Novel Way to Look at the Problem Space. In: Yin, H., et al. Intelligent Data Engineering and Automated Learning – IDEAL 2021. IDEAL 2021. Lecture Notes in Computer Science, vol 13113. Springer, Cham. https://doi.org/10.1007/978-3-030-91608-4_33
9. Hussain S, Ali M, Naseem U, Nezhadmoghadam F, Jatoi MA, Gulliver TA and Tamez-Peña JG (2024). Breast cancer risk prediction using machine learning: a systematic review. Front. Oncol. 14:1343627. https://doi.org/10.3389/fonc.2024.1343627
10. Srivastava, N., Hinton, G., Krizhevsky, A., Sutskever, I., & Salakhutdinov, R. (2014). Dropout: A simple way to prevent neural networks from overfitting. Journal of Machine Learning Research, 15(1), 1929-1958. https://dl.acm.org/doi/abs/10.5555/2627435.2670313
11. Angelone, F.; Ponsiglione, A.M.; Ricciardi, C.; Belfiore, M.P.; Gatta, G.; Grassi, R.; Amato, F.; Sansone, M. (2024). A Machine Learning Approach for Breast Cancer Risk Prediction in Digital Mammography. Appl. Sci. 2024, 14, 10315. https://doi.org/10.3390/app142210315
12. National Cancer Institute. (2020). SEER Cancer Statistics Review. Retrieved from https://seer.cancer.gov
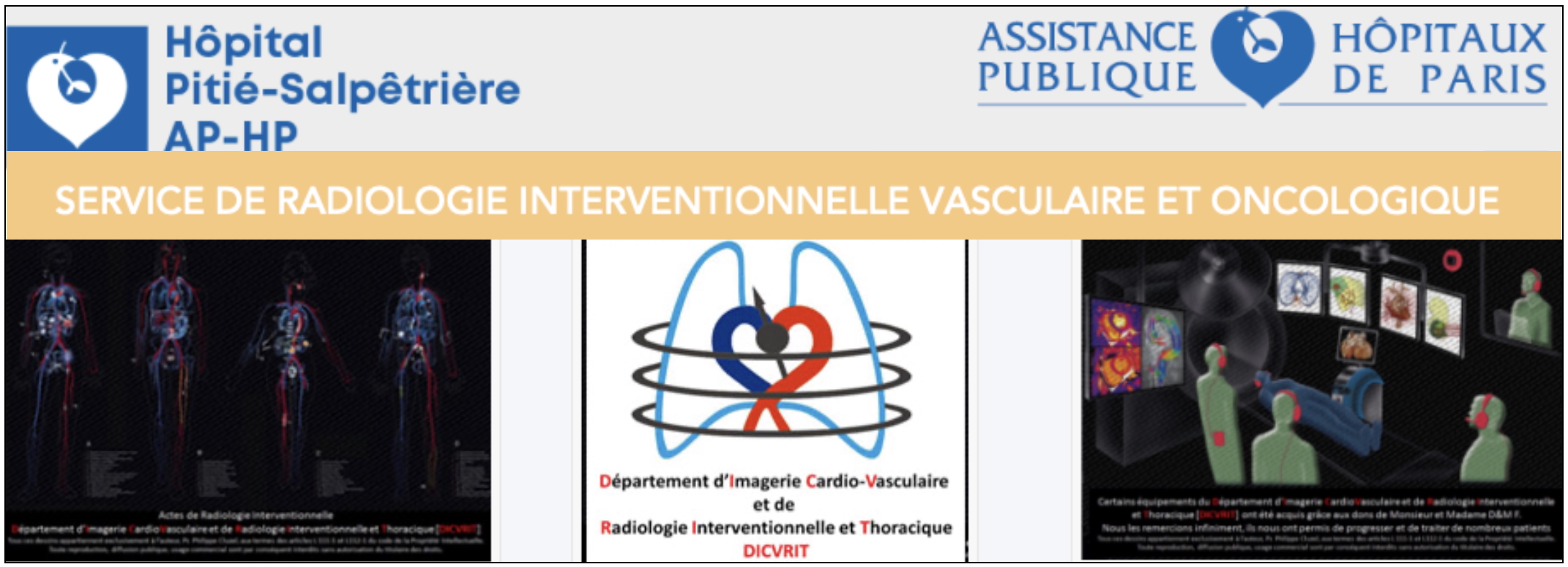
Downloads
Published
Issue
Section
License
Copyright (c) 2025 Dr. Imene Bouharati, Dr. Khaoula Bouharati

This work is licensed under a Creative Commons Attribution 4.0 International License.
This license requires that reusers give credit to the creator. It allows reusers to distribute, remix, adapt, and build upon the material in any medium or format, even for commercial purposes.